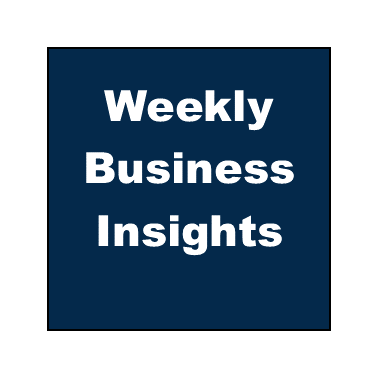
Extractive summaries of and key takeaways from the articles curated from TOP TEN BUSINESS MAGAZINES to promote informed business decision-making | Week 295 | May 5-11, 2023
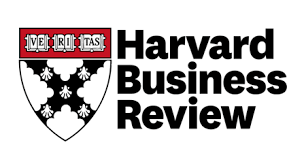
Analytics for Marketers
By Fabrizio Fantini and Das Narayandas | Harvard Business Review Magazine | May–June 2023 Issue
Listen to the Extractive Summary of the Article
Advanced analytics can help companies solve a host of management problems, including those related to marketing, sales, and supply-chain operations, which can lead to a sustainable competitive advantage. But as more data becomes available and advanced analytics are further refined, managers may struggle with when, where, and how much to incorporate machines into their business analytics, and to what extent they should bring their own judgment to bear when making data-driven decisions.
In general, humans are more capable in the areas of intuition and ambiguity resolution; machines are far superior at deduction, granularity, and scalability. How can you find the right balance? Three approaches:
- In descriptive analytics—commonly termed “business intelligence”—managers use machines to make sense of patterns in historical data. They essentially ask, “Help me understand what happened.” Managers provided with business-intelligence tools rely on past experience and high-level pattern recognition to project the past into the future, often relying on their gut. That can lead to repeating time-trusted approaches to solving problems rather than finding innovative new paths.
- With predictive analytics, machines determine the likely outcome or outcomes of a particular situation for different combinations of input variables, giving managers insight to choose the course of action whose expected result best meets their objective. These predictions allow managers to drill down and make decisions at the transactional and tactical levels as opposed to the typically high level of descriptive analytics. The predictive analytics approach is structurally limited. It’s nearly impossible to predict future demand (let alone the future itself) with much certainty.
- With prescriptive analytics, machines make decisions that are based on managers’ defined objectives, by employing large amounts of data to rapidly analyze market conditions and learn by designing and running large numbers of low-cost experiments and what-if scenarios.
Which approach to use in a given situation depends on two factors: the relevance of the available data and the strength of the business case. A successful balance between human and machine maximizes the contribution of each. When available data is limited and high levels of uncertainty exist, descriptive analytics is the most viable option for providing directional guidance to managers. As the frequency of decision-making increases, more granular data becomes available, and the relevance of the data to the problem increases, more-autonomous prescriptive analytics approaches tend to perform best. In intermediate cases, where only limited relevant data is available, a predictive analytics approach is preferred.
Business case: The profit-improvement potential derives from the amount of inefficiency that data-driven insights can be expected to address. But inefficiency isn’t a characteristic of every business problem. And when it is an issue it may be addressable only with data that is not readily available. Therefore, not all problems are amenable to advanced approaches.
3 key takeaways from the article
- There are three common approaches to marketing analytics: descriptive, focusing on understanding what happened; predictive, what will happen – where machines determine likely outcomes but humans choose which course to follow; and prescriptive, seeking guidance from machines on what should I do now.
- Which approach to use in a given situation depends on two factors: the relevance of the available data and the strength of the business case. A successful balance between human and machine maximizes the contribution of each.
- Humans and machines excel at different tasks: humans at dealing with limited data and applying intuition in unfamiliar contexts, and machines at making decisions, however granular and sparse, that are repeated in time or space or both, and in environments flooded with rich data.
(Copyright)
Topics: Technology, Decision-making, Data
Leave a Reply
You must be logged in to post a comment.