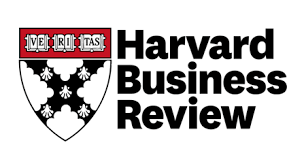
Extractive summaries and key takeaways from the articles curated from TOP TEN BUSINESS MAGAZINES to promote informed business decision-making | Since 2017 | Week 367 | September 20-26, 2024 | Archive
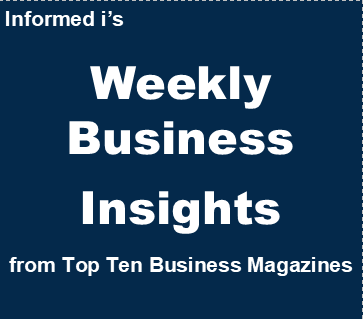
Where Data-Driven Decision-Making Can Go Wrong
By Michael Luca and Amy C. Edmondson | Harvard Business Review Magazine | September–October 2024 Issue
Extractive Summary of the Article | Listen
Whether evidence comes from an outside study or internal data, walking through it thoroughly before making major decisions is crucial. Too often predetermined beliefs, problematic comparisons, and groupthink dominate discussions. Five common pitfalls leaders make in interpreting analyses are:
- Pressure-Test the Link Between Cause and Effect. Will search engine advertisements increase sales? Will allowing employees to work remotely reduce turnover? These questions are about cause and effect—and are the kind of questions that data analytics can help answer. In fact, research papers have looked at them in detail. However, managers frequently misinterpret how the findings of those and other studies apply to their own business situation. When making decisions, managers should consider internal validity—whether an analysis accurately answers a question in the context in which it was studied. They should also consider external validity—the extent to which they can generalize results from one context to another. That will help them avoid making five common mistakes:
- Conflating causation with correlation. Even though most people know that correlation doesn’t equal causation, this error is surprisingly prevalent. To understand causality, delve into how the study in question was conducted. For instance, was it a randomized controlled trial, in which the researchers randomly assigned people to two groups: one that was subjected to a test condition and a control group that was not? That’s often considered the gold standard for assessing cause and effect, though such experiments aren’t always feasible or practical. Researchers who don’t have access to planned or natural experiments may instead control for potential confounding factors—variables that affect the variable of interest—in their data analysis, though this can be challenging in practice.
- Underestimating the importance of sample size. Small sample sizes are more likely to show greater fluctuations. Psychologists Daniel Kahneman and Amos Tversky, in their canonical work on biases and heuristics, found that most people got the answer wrong, with more than half saying, “About the same.” People tend to underappreciate the effect that sample size has on the precision of an estimate. This common error can lead to bad decisions. When evaluating effects, it can be helpful to ask not only about the sample size but about the confidence interval.
- Focusing on the wrong outcomes. In their classic 1992 HBR article “The Balanced Scorecard: Measures That Drive Performance,” Robert S. Kaplan and David P. Norton opened with a simple observation: “What you measure is what you get.” Although their article predates the era of modern analytics, that idea is more apt than ever. Experiments and predictive analytics often focus on outcomes that are easy to measure rather than on those that business leaders truly care about but are difficult or impractical to ascertain. As a result, outcome metrics often don’t fully capture broader performance in company operations. It’s also important to make sure that the outcome being studied is a good proxy for the actual organizational goal in question. Some company experiments track results for just a few days and assume that they’re robust evidence of what the longer-term effect would be. With certain questions and contexts, a short time frame may not be sufficient.
- To really learn from any data set, you need to ask basic questions like, What outcomes were measured, and did we include all that are relevant to the decision we have to make? Were they broad enough to capture key intended and unintended consequences? Were they tracked for an appropriate period of time?
- Misjudging generalizability. Business leaders make missteps in both directions, either over- or underestimating the generalizability of findings. When you’re assessing generalizability, it can be helpful to discuss the mechanisms that might explain the results and whether they apply in other contexts. You might ask things like, How similar is the setting of this study to that of our business? Does the context or period of the analysis make it more or less relevant to our decision? What is the composition of the sample being studied, and how does it influence the applicability of the results? Does the effect vary across subgroups?
- Overweighting a specific result. Relying on a single empirical finding without a systematic discussion of it can be just as unwise as dismissing the evidence as irrelevant to your situation. It’s worth checking for additional research on the subject. Conducting an experiment or further analysis with your own organization can be another good option. Questions to ask include, Are there other analyses that validate the results and the approach? What additional data might we collect, and would the benefit of gathering more evidence outweigh the cost of that effort?
To overcome bias, business leaders can invite contributors with diverse perspectives to a conversation, ask them to challenge and build on ideas, and ensure that discussions are probing and draw on high-quality data. Encouraging dissent and constructive criticism can help combat groupthink, make it easier to anticipate unintended consequences, and help teams avoid giving too much weight to leaders’ opinions. Leaders also must push people to consider the impact of decisions on various stakeholders and deliberately break out of siloed perspectives.
3 key takeaways from the article
- Whether evidence comes from an outside study or internal data, walking through it thoroughly before making major decisions is crucial. Too often predetermined beliefs, problematic comparisons, and groupthink dominate discussions.
- Five common pitfalls leaders make in interpreting analyses are: Pressure-Test the Link Between Cause and Effect, Conflating causation with correlation, Underestimating the importance of sample size, Focusing on the wrong outcomes, Misjudging generalizability, and Overweighting a specific result.
- To overcome bias, business leaders can invite contributors with diverse perspectives to a conversation, ask them to challenge and build on ideas, and ensure that discussions are probing and draw on high-quality data. Encouraging dissent and constructive criticism can help combat groupthink, make it easier to anticipate unintended consequences, and help teams avoid giving too much weight to leaders’ opinions. Leaders also must push people to consider the impact of decisions on various stakeholders and deliberately break out of siloed perspectives.
(Copyright lies with the publisher)
Topics: Decision-making, Uncertainty, Critcial Thinking, Biases, Data, Research
Leave a Reply
You must be logged in to post a comment.