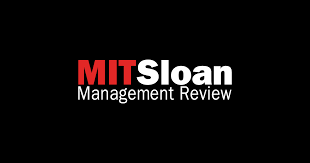
Weekly Business Insights from Top Ten Business Magazines | Week 328
Extractive summaries and key takeaways from the articles curated from TOP TEN BUSINESS MAGAZINES to promote informed business decision-making | Since September 2017 | Week 328 | December 22-28, 2023
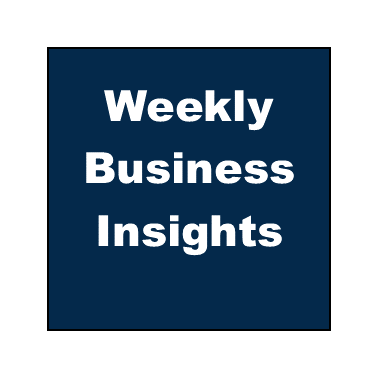
Personal Development, Leadership & Managing | Section 2
What Managers Should Ask About AI Models and Data Sets
By Roger W. Hoerl and Thomas C. Redman | MIT Sloan Management Review | December 19, 2023
Extractive Summary of the Article | Listen
The power of AI and the machine learning models on which it is based continue to reshape the rules of business. However, too many AI projects are failing — often after deployment, which is especially costly and embarrassing. Just ask Amazon about its facial recognition fiascos, or Microsoft about its blunders with its Tay chatbot. Too often, data scientists write off such failures as individual anomalies without looking for patterns that could help prevent future failures. Today’s senior business managers have the power — and the responsibility — to prevent post-deployment failures. But to do so, they must understand more about the data sets and data models in order to both ask the right questions of AI model developers and evaluate the answers.
An AI project’s success or failure depends on the data set it uses. To help teams get to the right data, the authors offer a five-element framework:
- Business problem: a clear statement of the problem needs to be solved
- The concept of the right data: is this the right data to solve the problem at hand
- The training data: the data actually used to train the AI
- The model/algorithm: the result of the machine learning exercise
- Future data: the data you don’t have yet
With this background, managers should ask a sequence of pointed questions at three key stages, based on the Right Data Framework.
- Assuming this project is successful, how and where do you anticipate the models that you develop will be utilized?
- How will you acquire training data that meets the right data criteria?
- What steps have you taken to understand the full history, subtlety, advantages, and limitations of the training data acquired? How does it compare with the right data criteria?
- How will you check that future data meets the right data criteria?
- How will you ensure that future data meets your expectations? What controls for both data and models are in place to ensure both successful deployment and model accuracy with future data?
- What are the top three ways you could envision your models failing in deployment? What steps have you taken to mitigate them?
3 key takeaways from the article
- The power of AI and the machine learning models on which it is based continue to reshape the rules of business. However, too many AI projects are failing — often after deployment, which is especially costly and embarrassing.
- Today’s senior business managers have the power — and the responsibility — to prevent post-deployment failures. But to do so, they must understand more about the data sets and data models in order to both ask the right questions of AI model developers and evaluate the answers.
- A five-element framework can help: Business problem: a clear statement of the problem needs to be solved; The concept of the right data: is this the right data to solve the problem at hand; The training data: the data actually used to train the AI; The model/algorithm: the result of the machine learning exercise; and Future data: the data you don’t have yet.
(Copyright lies with the publisher)
Topics: Technology, Artificial Intelligence, Project Management
Leave a Reply
You must be logged in to post a comment.