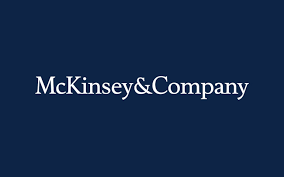
Weekly Business Insights from Top Ten Business Magazines
Extractive summaries and key takeaways from the articles curated from TOP TEN BUSINESS MAGAZINES to promote informed business decision-making | Since September 2017 | Week 350 | May 24-30, 2024
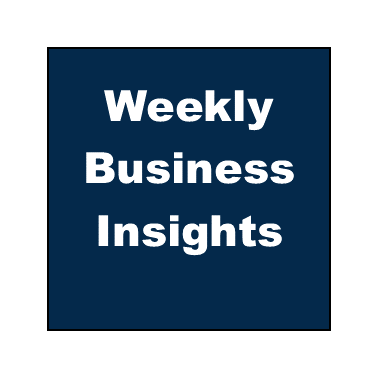
Strategy & Business Model Section | 2
Moving past gen AI’s honeymoon phase: Seven hard truths for CIOs to get from pilot to scale
By Aamer Baig et al., | McKinsey & Company | May 13, 2024
Extractive Summary of the Article | Read and/or Listen
The honeymoon phase of generative AI (gen AI) is over. As most organizations are learning, it is relatively easy to build gee-whiz gen AI pilots, but turning them into at-scale capabilities is another story. The difficulty in making that leap goes a long way to explaining why just 11 percent of companies have adopted gen AI at scale. This maturing phase is a welcome development because it gives CIOs an opportunity to turn gen AI’s promise into business value. Yet while most CIOs know that pilots don’t reflect real-world scenarios—that’s not really the point of a pilot, after all—they often underestimate the amount of work that needs to be done to get gen AI production ready. Ultimately, getting the full value from gen AI requires companies to rewire how they work, and putting in place a scalable technology foundation is a key part of that process.
Seven things that Shapers need to know and do – an approach in which companies develop a competitive advantage by connecting large language models (LLMs) to internal applications and data sources:
- Eliminate the noise, and focus on the signal. Be honest about what pilots have worked. Cut down on experiments. Direct your efforts toward solving important business problems.
- It’s about how the pieces fit together, not the pieces themselves. Too much time is spent assessing individual components of a gen AI engine. Much more consequential is figuring out how they work together securely.
- Get a handle on costs before they sink you. Models account for only about 15 percent of the overall cost of gen AI applications. Understand where the costs lurk, and apply the right tools and capabilities to rein them in.
- Tame the proliferation of tools and tech. The proliferation of infrastructures, LLMs, and tools has made scaled rollouts unfeasible. Narrow down to those capabilities that best serve the business, and take advantage of available cloud services (while preserving your flexibility).
- Create teams that can build value, not just models. Getting to scale requires a team with a broad cross-section of skills to not only build gen AI applications but also make sure they generate the value they’re supposed to, safely and securely.
- Go for the right data, not the perfect data. Targeting which data matters most and investing in its management over time has a big impact on how quickly you can scale.
- Reuse it or lose it. Reusable code can increase the development speed of generative AI use cases by 30 to 50 percent.
3 key takeaways from the article
- The honeymoon phase of generative AI (gen AI) is over. As most organizations are learning, it is relatively easy to build gee-whiz gen AI pilots, but turning them into at-scale capabilities is another story.
- Getting the full value from gen AI requires companies to rewire how they work, and putting in place a scalable technology foundation is a key part of that process.
- Seven things that Shapers need to know and do – an approach in which companies develop a competitive advantage by connecting large language models (LLMs) to internal applications and data sources: eliminate the noise, and focus on the signal; it’s about how the pieces fit together, not the pieces themselves; get a handle on costs before they sink you; tame the proliferation of tools and tech; create teams that can build value, not just models; go for the right data, not the perfect data; and reuse it or lose it.
(Copyright lies with the publisher)
Topics: Technology, Artificial Intelligence, Productivity, Chief Technology Officers
Leave a Reply
You must be logged in to post a comment.