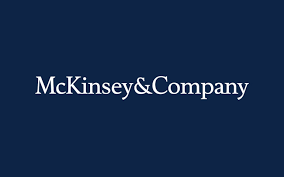
Strategy & Business Model Section | 1
Extractive summaries and key takeaways from the articles curated from TOP TEN BUSINESS MAGAZINES to promote informed business decision-making | Since September 2017 | Week 326 | December 8-14, 2023
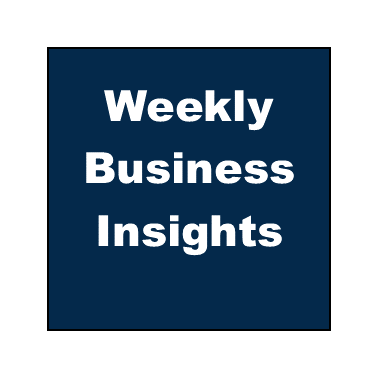
Capturing the full value of generative AI in banking
By Vishnu Kamalnath et al., | McKinsey & Company | December 5, 2023
Extractive Summary of the Article | Listen
Generative AI (gen AI) burst onto the scene in early 2023 and is showing clearly positive results—and raising new potential risks—for organizations worldwide. Banking leaders appear to be on board, even with the possible complications.
The McKinsey Global Institute estimates that among industries globally, gen AI could add the equivalent of $2.6 trillion to $4.4 trillion annually in value across the 63 use cases it analyzed. Among industry sectors, banking is expected to have one of the largest opportunities: an annual potential of $200 billion to $340 billion (equivalent to 9 to 15 percent of operating profits), largely from increased productivity. The economic impact will likely benefit all banking segments and functions, with the greatest absolute gains in the corporate and retail sectors ($56 billion and $54 billion, respectively. Notably, while banks have rightly focused on productivity in their initial gen AI pilots due to the broader pressure on banking economics, the technology could greatly alter how some jobs are done and how customers interact with banks. It might even lead to entirely new business models.
Several factors explain why scaling gen AI is different. The first is the scope of the task and related implications. Just as the smartphone catalyzed an entire ecosystem of businesses and business models, gen AI is making relevant the full range of advanced analytics capabilities and applications. The second factor is that scaling gen AI complicates an operating dynamic that had been nearly resolved for most financial institutions. Third, the pace of change has never been faster. Finally, scaling up gen AI has unique talent-related challenges, whose magnitude will depend greatly on a bank’s talent base.
The authors found that delivering sustained value, beyond initial proofs of concept, requires strong capabilities across seven dimensions. Management teams with early success in scaling gen AI have started with a strategic view of where gen AI, AI, and advanced analytics more broadly could play a role in their business. A sustained approach for an evolving set of required skills and capabilities. An operating model that is fit for scale-up involving cross-functional teams and alignment of accountabilities and responsibilities between delivery and business teams. Early successes in scaling gen AI occurred when banks carefully weighed the “build versus buy versus partner” options for their technology needs. Leading banks are using a combination of human talent and automation. Interven at multiple points in the data life cycle to ensure quality of all data. Banks need to redesign their risk- and model-governance frameworks and develop new sets of controls. And a successful gen AI scale-up also requires a comprehensive change management plan.
2 key takeaways from the article
- Generative AI (gen AI) burst onto the scene in early 2023 and is showing clearly positive results—and raising new potential risks—for organizations worldwide. For banks seeking to tap this valuable technology, a gen AI scale-up is like nothing most leaders have ever seen.
- The authors found that delivering sustained value, beyond initial proofs of concept, requires strong capabilities across seven dimensions: start with a strategic view of where gen AI, AI, and advanced analytics more broadly could play a role in their business; bring required skills and capabilities; involve cross-funcaiontal teams; carefully weighed the “build versus buy versus partner” options for their technology needs; using a combination of human talent and automation; intervene at multiple points in the data life cycle to ensure quality of all data; redesign their risk- and model-governance frameworks and develop new sets of controls; and follow a change management plan.
(Copyright lies with the publisher)
Topics: Technology, Artificial Intelligence, Banking, Strategy, Business Model
Leave a Reply
You must be logged in to post a comment.